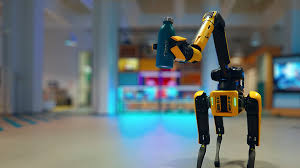
Embodied Artificial Intelligence (AI) represents a significant paradigm shift in the field of AI research. Unlike traditional AI, which primarily operates in the digital realm, Embodied AI integrates physical entities, such as robots, to interact dynamically with the real world. This approach aims to enhance the capabilities of AI systems by leveraging physical interactions to develop more sophisticated and contextually aware intelligence. This report delves into the current state of Embodied AI, its challenges, advancements, and future directions, drawing on various sources and research studies.
1 THE CONCEPT OF EMBODIED AI
Embodied AI places the physical entity of the body at the center of the research subject to gain deeper insights into intelligence. It explores how the brain and the body as a whole develop through physical interactions with the real world. This approach contrasts with traditional AI, which often relies on static data sets and lacks the ability to interact with and adapt to dynamic environments [1].
2 HISTORICAL CONTEXT AND EVOLUTION
The concept of embodiment in AI has evolved over several decades, drawing from diverse fields such as philosophy, psychology, neuroscience, and robotics. The shift towards embodiment marks a departure from the classical paradigm of static learning, emphasizing the importance of physical interaction for contextual understanding and adaptive learning [2].
3 KEY COMPONENTS AND FRAMEWORKS
Embodied AI systems are characterized by several essential components, including perception, action, memory, and learning. These components are integrated into cognitive architectures that enable AI agents to interact with their environments in a manner similar to humans. The framework for Embodied AI aligns with Friston’s active inference principle, which emphasizes the role of perception and action in learning and decision-making [2].
4 ADVANCEMENTS IN EMBODIED AI
4.1 Autonomous Manipulation and Planning
Recent advances in computer vision, natural language processing, and multi- modality learning have significantly enhanced the capabilities of Embodied AI systems. Foundation models have demonstrated superhuman capabilities for specific tasks, providing a solid cornerstone for integrating basic modules into Embodied AI systems. These advancements have shown promise in autonomous manipulation, high-level planning, and low-level control [3].
4.2 Benchmarks and Datasets
The development of comprehensive benchmarks and datasets is crucial for evaluating the performance of Embodied AI systems. Stanford University’s BEHAVIOR benchmark, for instance, includes 100 everyday household activities designed to reproduce real-world challenges. This benchmark provides a set of metrics to quantify the performance of Embodied AI, including success scores and efficiency metrics [4].
4.3 Educational Applications
Embodied AI has also found applications in education, particularly in enhancing language learning and self-directed learning using mobile technology. AI chatbots and other tools have been developed to assist in second language acquisition, providing personalized and interactive learning experiences [5].
5 CHALLENGES AND LIMITATIONS
5.1 Data Bottleneck
One of the significant challenges in Embodied AI is the data bottleneck. The development and refinement of Embodied AI systems heavily rely on extensive, high-quality datasets.
However, procuring data from interactions between robots and their operating environments is a daunting task due to the variety and complexity of these environments [6].
5.2 Computing Architecture
Current computational frameworks are often inadequate for the intricate demands of Embodied AI. The need for real-time processing of vast data streams, high concurrency, reliability, and energy efficiency poses significant challenges. Innovative computing architectures tailored to the nuanced requirements of Embodied AI are essential for overcoming these limitations [6].
5.3 Sensor Integration and Real-Time Processing
Developing and deploying Embodied AI systems also involve challenges related to sensor integration, real-time processing, and human-machine interface design. These challenges must be addressed to improve the efficacy of AI systems in dynamic and unpredictable environments [7].
6 FUTURE DIRECTIONS
6.1 Interdisciplinary Collaboration
Advancing Embodied AI requires interdisciplinary collaboration, bringing together expertise from fields such as biology, neuroscience, engineering, robotics, biomechanics, material sciences, and dynamical systems. This transdisciplinary approach is essential for addressing the complex challenges and seizing the opportunities in Embodied AI research [8].
6.2 Standardized Frameworks
The development of standardized frameworks for Embodied AI is crucial for ensuring consistency and comparability across different research studies and applications. These frameworks should encompass cognitive architectures, sensor and actuator technologies, and evaluation metrics [7].
6.3 Investment in Advanced Hardware
Substantial investment in advanced hardware is necessary to support the computational demands of Embodied AI. This includes developing innovative computing architectures and sensor technologies that can handle the realtime processing and high concurrency required for dynamic environments [2].
6.4 Ethical and Social Implications
As Embodied AI continues to evolve, it is essential to consider the ethical and social implications of deploying intelligent agents in real-world environments. This includes addressing issues related to privacy, security, and the potential impact on employment and human-machine interactions [1].
7 CONCLUSIONS
Embodied AI represents a transformative approach to artificial intelligence, emphasizing the importance of physical interaction for developing more sophisticated and contextually aware intelligence. While significant advancements have been made, numerous challenges remain, including data bottlenecks, inadequate computing architectures, and sensor integration. Addressing these challenges requires interdisciplinary collaboration, investment in advanced hardware, and the development of standardized frameworks. As the field continues to evolve, it is crucial to consider the ethical and social implications of deploying Embodied AI systems in real-world environments.
REFERENCES
[1] M. T. Shahria, M. S. H. Sunny, M. I. I. Zarif, J. Ghommam, S. I. Ahamed, and M. H. Rahman, “A Comprehensive Review of Vision-Based Robotic Applications: Current State, Components, Approaches, Barriers, and Potential Solutions,” Robotics, vol. 11, no. 6, p. 139, Dec. 2022. [Online]. Available: https://www.mdpi.com/2218-6581/11/6/139
[2] G. Paolo, J. Gonzalez-Billandon, and B. Kégl, “A call for embodied AI,” Jul. 2024. [Online]. Available: http://arxiv.org/abs/2402.03824
[3] Z. Xu, K. Wu, J. Wen, J. Li, N. Liu, Z. Che, and J. Tang, “A Survey on Robotics with Foundation Models: Toward Embodied AI,” Feb. 2024. [Online]. Available: http://arxiv.org/abs/2402.02385
[4] “Stanford Releases New Benchmarks for Embodied AI | Psychology Today.” [Online]. Available:https://www.psychologytoday.com/intl/blog/the-future-brain/202109/stanford-releases-new-benchmarks-embodied-ai [5] B. Memarian and T. Doleck, “Embodied AI in education: A review on the body, environment, and mind,” Education and Information Technologies, vol. 29, no. 1, pp. 895–916, Nov. 2023. [Online]. Available: https://doi.org/10.1007/s10639-023-12346-8 [6] “Building Computing Systems for Embodied Artificial Intelligence – Communications of the ACM,” Mar.
2024. [Online]. Available: https://cacm.acm.org/blogcacm/building-computing-systems-for-embodied artificial-intelligence/
[7] E. Idowu, “Bridging the Embodiment Gap: Embodied AI for Enhanced Human-Machine Collaboration and Learning in Dynamic Environments,” Jul. 2024. [Online]. Available: https://www.preprints.org/manuscript/202407.0869/v1 [8] R. Pfeifer and F. Iida, “Embodied Artificial Intelligence: Trends and Challenges,” in Embodied Artificial Intelligence, D. Hutchison, T. Kanade, J. Kittler, J. M. Kleinberg, F. Mattern, J. C. Mitchell, M. Naor, O. Nierstrasz, C. Pandu Rangan, B. Steffen, M. Sudan, D. Terzopoulos, D. Tygar, M. Y. Vardi, G. Weikum, F. Iida, R. Pfeifer, L. Steels, and Y. Kuniyoshi, Eds. Berlin, Heidelberg: Springer Berlin Heidelberg, 2004, vol. 3139, pp. 1–26. [Online]. Available: http://link.springer.com/10.1007/978-3-540-27833-7_1